Published
- 9 min read
Collectionless Artificial Intelligence
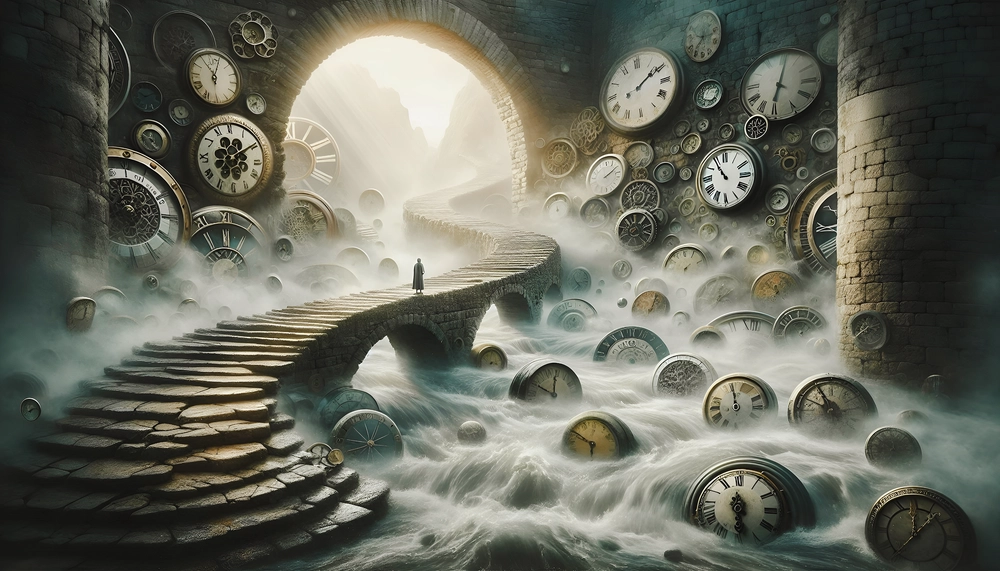
Beyond Mainstream AI
Learning from huge data collections introduces risks related to data centralization, privacy, energy efficiency, limited customizability, and control. Collectionless AI focusses on the perspective in which artificial agents are progressively developed over time by online learning from potentially lifelong streams of sensory data. This is achieved without storing the sensory information and without building datasets for offline-learning purposes while pushing towards interactions with the environment, including humans and other artificial agents.
- Marco Gori & Stefano Melacci; from the Research Summary published by the Montreal AI Ethics Institute (MAIEI) Link
- See the draft with the main ideas behind Collectionless AI: https://arxiv.org/abs/2309.06938 [Paper by: Marco Gori, Stefano Melacci]
- Also have a look at this paper (NeurIPS Data-Centric AI Workshop 2021): Can machines learn to see without visual databases? [Paper by: Alessandro Betti, Marco Gori, Stefano Melacci, Marcello Pelillo, Fabio Roli]
Here is a video about the paper above (warning: 2021, we were not using the keyword Collectionless AI yet). Have a look at it! (in the video: Stefano Melacci - yes, my hair is now white/light-gray :))
What is Collectionless AI?
Collectionless AI is a radically different perspective on AI technologies, which emerges as we think of machines that acquire cognitive skills without accessing previously stored data collections but simply by environmental interactions, where the sensory information is immediately processed (learning online), and agent-to-human or agent-to-agent exchanges occur.
In nature, animals do not rely on data collections but process information as time passes and create (and update) an appropriate internal representation of the environment. It is the interaction with the environment that allows them to be in touch with the treasure of information, which enables the growth of their cognitive skills. Artificial neural networks are still struggling to find a good trade-off between plasticity and stability without relying on optimizing previously built data collections (including data replays or knowledge distillation from previously trained models), which is the main focus of Collectionless AI.
Time as the protagonist of learning
Sensory information is characterized by a natural temporal development of the data that is commonly neglected by current technologies. In nature, we do not learn from a huge dataset of “shuffled images”, and we do not store our entire visual life, stochastically sampling from it.
Why can they afford to see it without accessing a previously stored database? Is there a specific biological aspect that cannot be captured in machines? Collectionless AI sustains the position that machines can likely gain those skills, once we face the challenge of learning without using data collections, exploiting the natural development of the sensory information over time.
Is there a problem with current AI technologies?
The growing ubiquity of Large Language Models (LLM) has recently opened strong debates on scenarios giving rise to potentially rogue AIs involving social and political aspects. The source of these debates is deeply connected with the exploitation of increasingly large data collections, which requires huge financial resources, thus leading to the centralization of information. This aspect produces undeniable privacy problems as well as very controversial geopolitical effects. In a nutshell:
- Data centralization issues
- Privacy and geopolitical issues
- Energy efficiency issues
- Limited control, customizability, and causality
On-device learning, without building databases, might become an important requirement of future AI-based technologies. When pushing collection-centered AI, we implicitly contribute to creating serious geopolitical issues connected with the domain of a few countries that can control data and the development of technologies exploiting them
Should I get rid of datasets?
NO! Collectionless AI remarks the importance of opening to a different perspective when developing AI technologies, and NOT to forget about what we currently already have in our hands. If datasets are there, then why not use them?
The outstanding results of current Machine Learning-based models should be still massively leveraged by the current technologies. They are all based on previously collected datasets and networks trained by stochastically sampling from them, without any dynamic human intervention: humans are only bare data labelers, then they are out of the game. If we want to adapt a previously trained model to different conditions, or if we want to correct its predictions by interacting with it, we simply cannot, unless we go back to the data collection process, and learn from it. This dataset-centric perspective is leading to great results, but it is not flexible, the current models are not easily adaptable to new conditions, they learn from dataset with redundant information, being them extremely greedy and with low sample efficiency.
Collectionless AI pushes toward the direction of developing models that can be adapted over time, that can work in new conditions without restarting the whole learning process, that can be corrected on the fly, that can do the most of the information they receive, abandoning the idea of doing everything by statistically learning from previously collected data.
Am I prohibited from retaining data?
You can store whatever you like! However, consider that it does not make much sense to store all the processed data or to make the storage capacity a function of time without any realistic upper-bounds! Recall that we are talking about agents that might live forever.
Collectionless AI remarks the importance of learning online, continually, doing the most out of what is available at the current instant of the agent’s life. Of course, it is an extreme point of a direction that can be followed by performing steps of different lengths.
Why on-device computation?
We have the use of smart devices that are in our hands everyday (smartphones). These devices can continuously collect information from the surrounding environments, they can communicate with you and with other devices. Going beyond this example, can we think of a learning mechanism that favors the role of on-the-edge devices and that can be controlled in the way it shares information with other devices?
Collectioless AI suggests that we have to think about agents that run in a local sandbox we can fully control (local device), and it promotes the idea of devising mechanisms to let agents interact with humans and other changes, instead of simply storing datasets and sending them to others. This suggests the need of doing the most of the interactions, remarking the importance of such a long-standing challenge.
How can I evaluate my model?
One of the most controversial aspects of mainstream Machine Learning research is about “benchmarks”. While many interesting approaches are shown to improve the accuracy over popular benchmarks, their performance is not evaluated “in the wild”. Benchmarks are definitely needed to measure progress of machines that learn over time, exactly like exams are used to evaluate the knowledge of students on a specific subject. However, it is by interacting with students that we can understand more about their knowledge! Think about a written exam and an oral one. Some students are able to do a good job in some written tests that might be related to the ones provided the previous year, but when it comes to an oral examination, their “overfitting” becomes evident.
Just like humans, machines can also be expected to “live in their environment”, and they can be evaluated online. A massive online active evaluation open to a wide audience can be a viable path for qualitatively evaluating virtual agents that progressively learn, as in the case of LLMs. The quality of the same agent can be evaluated at different stages of its evolution, analyzing progress and regressions.
I see connections with Reinforcement Learning, Continual Learning, and others
Yes of course, that’s kind of obvious. Collectionless AI is not another learning setting which is orthogonal to the existing ones. It is a perspective to drive the studies on the next-gen AI technologies. Since it is about exploring, interacting, learning online, lifelong learning, etc… it includes a lot of largely known topics from existing literature.
Can we go beyond the boundaries of the common topics we consider in research activities? Let’s think about a perspective, an ambitious goal we would like to achieve. Existing topics and knowledge are definitely something we have to consider, but we should relax their “borders”, focussing on doing the most we can in the context which is remarked by Collectionless AI.
These things were already known, weren’t they?
What Collectionless AI suggests is a popular challenge of AI/ML, whenever we talk about mimicking human behavior. However, the mainstream of AI/ML went toward another direction, with outstanding results: collected data, learn, deploy, that’s it (and it works very very well!).
Let’s keep our eyes on what is not there yet. Companies can do the best with already existing technologies that are now very accessible. As researches, we have to look ahead, doing something more than changing yet-another block in a deep network and showing results that are better than the existing ones by a few decimals, shown in bold and in setups that are sometimes pretty custom or not fully specified. Let’s think about machines that live forever, that learn forever, adapt, improve. Let’s interact with them!
What do you expect from Collectionless AI?
Well, we hope that the scientific community will follow this perspective, driving their research along the direction suggested by Collectionless AI.
The extreme position promoted by Collectionless AI is intended to stimulate the development of new foundations on computational processes of learning and reasoning that might open the doors to a truly orthogonal competitive track on AI technologies that avoid data accumulation by design and that can be easily updated/adapted over time, thus offering a framework which is better suited concerning privacy issues, control and customizability.
Hey, what about Symbolic AI?
The largely-known big picture of Artificial Intelligence that emerges from Russel and Norvig is centered around a few classic topics, whose methodologies can, amongst others, be characterized by the noticeable difference that while “symbolic AI” is mostly collectionless, “sub-symbolic AI” strongly relies on large data collections. It is noteworthy that even symbolic approaches to AI are based on relevant collections of information, but in this case they are primarily knowledge bases, and there are no sensory data samples directly collected from the environment where the agent lives, thus at a different abstraction level.
In a sense, Collectionless AI is suggesting a route to reduce the distance between these two worlds.