Published
- 2 min read
INNS - Webinar Lecture on Cognidynamics
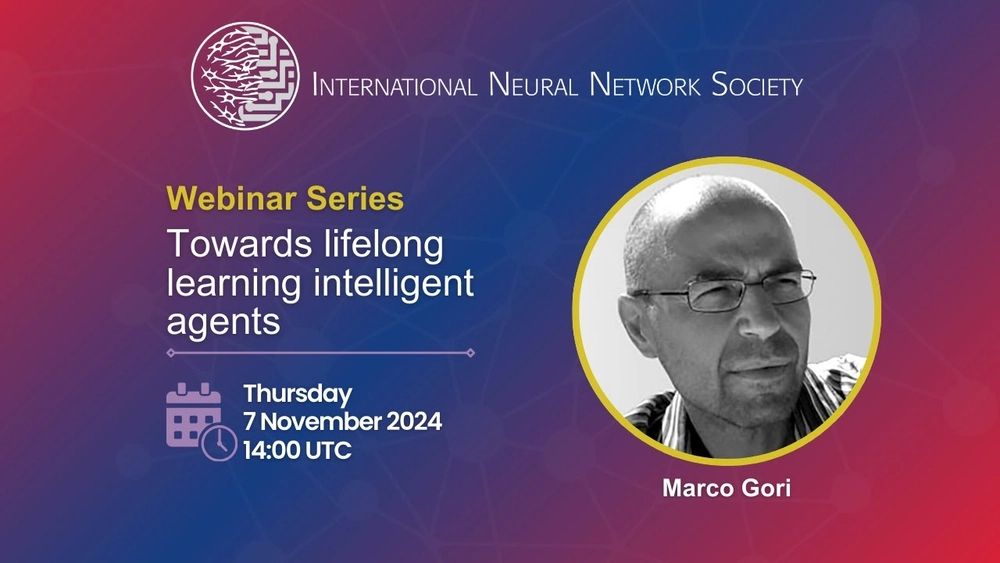
The fields of Artificial Intelligence (AI) and Cognitive Science began intersecting significantly during the Eighties when the Connectionist wave strongly propelled studies on Artificial Neural Networks. The evolution of AI over the last few decades, focusing on deep learning and, more recently, generative AI, has produced spectacular results that were hardly predictable even by the pioneers of the discipline. However, when examining early studies on Connectionism, many aspirations remain unrealized, as most successful outcomes rely on the brute force of combining computational resources with large data collections. This stands in contrast to nature, where cognition emerges from environmental interactions and the processing of temporal information. In order to capture those natural processes and explore an alternative path to Machine Learning, in this talk I introduce the framework of Cognidynamics that describes cognitive systems whose environmental interactions are driven by the minimization of a functional over time. This functional, referred to as cognitive action, replaces the traditional statistical functional risk of Machine Learning in the temporal dimension. I employ the tools of Theoretical Physics and Optimal Control to derive unified laws of cognition for learning and inference in recurrent neural networks. I demonstrate that Hamiltonian equations, in their causal dissipative form, lead to a novel neural propagation scheme that is local in both space and time. This addresses the longstanding debate on the biological plausibility of Backpropagation and offers a new framework for developing lifelong learning intelligent agents capable of focusing attention and taking conscious actions.